
To make things easy, install the Jupyter notebook and/or Jupyter lab: $ conda install -c conda-forge jupyter jupyterlab Next, let’s make sure everything went as expected.
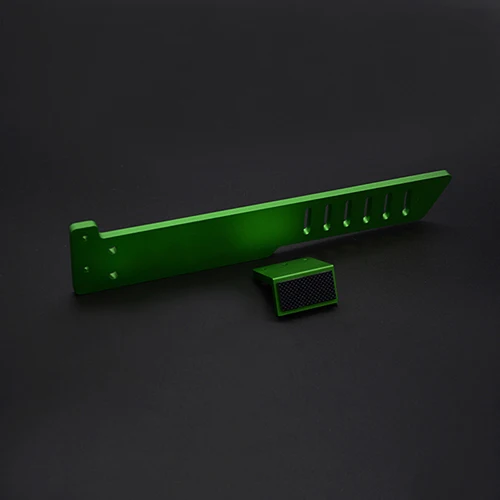
Note 1: Do not confuse Apple’s MPS (Metal Performance Shaders) with Nvidia’s MPS! ( Multi-Process Service). The MPS backend device maps machine learning computational graphs and primitives on the MPS Graph framework and tuned kernels provided by MPS.

Internally, PyTorch uses Apple’s M etal P erformance S haders (MPS) as a backend. PyTorch worked in conjunction with the Metal Engineering team to enable high-performance training on GPU. PyTorch, like Tensorflow, uses the Metal framework - Apple’s Graphics and Compute API. You can access all the articles in the “Setup Apple M-Silicon for Deep Learning” series from here, including the guide on how to install Tensorflow on Mac M1. PyTorch introduces GPU acceleration on M1 MacOS devices. Using the Metal plugin, Tensorflow can utilize the Macbook’s GPU. Tensorflow was the first framework to become available in Apple Silicon devices. Next on the agenda was compatibility with the popular ML frameworks. Hence, M1 Macbooks became suitable for deep learning tasks.
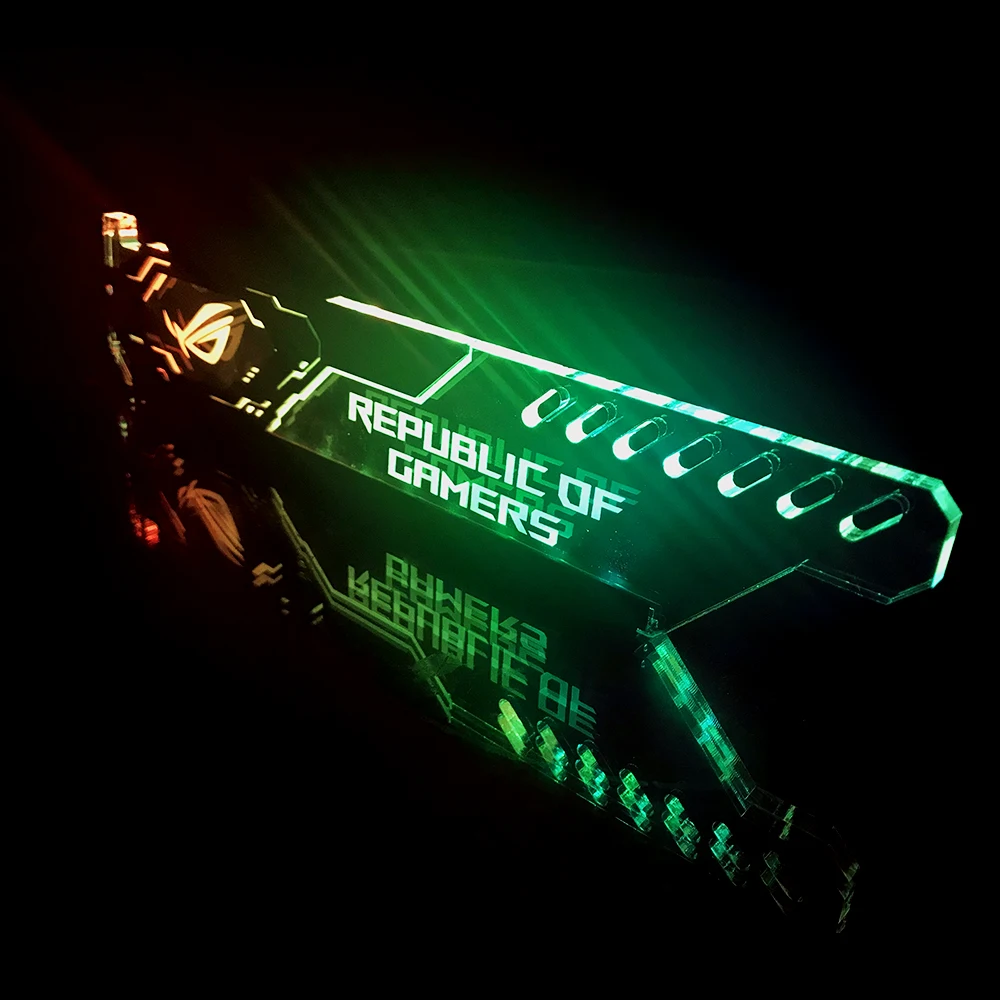
Starting with the M1 devices, Apple introduced a built-in graphics processor that enables GPU acceleration. The trajectory of Deep Learning support for the MacOS community has been amazing so far.
